
vegefox.com | stock.adobe.com
After several years, New York’s Packaging Reduction and Recycling Infrastructure Act looks like it might soon be voted into law. With support from several key environmental groups, as well as New York’s Department of Sanitation, there’s renewed hope that the bill will pass this year, helping the state reduce the four pounds a day of municipal solid waste it produces per capita and mostly sends to landfills or incinerators.
There’s also hope that once the legislation passes, it will inspire other states to follow suit. Extended Producer Responsibility (EPR) bills, which shift recycling responsibility from consumers to packaging producers, have already been passed in Maine, Colorado, Oregon, and California. The latest–in California–is one of the most aggressive laws passed to date, aiming to eliminate 25 percent of single-use plastic today while also improving the material’s recyclability in the packaging and food ware industries by 65 percent.
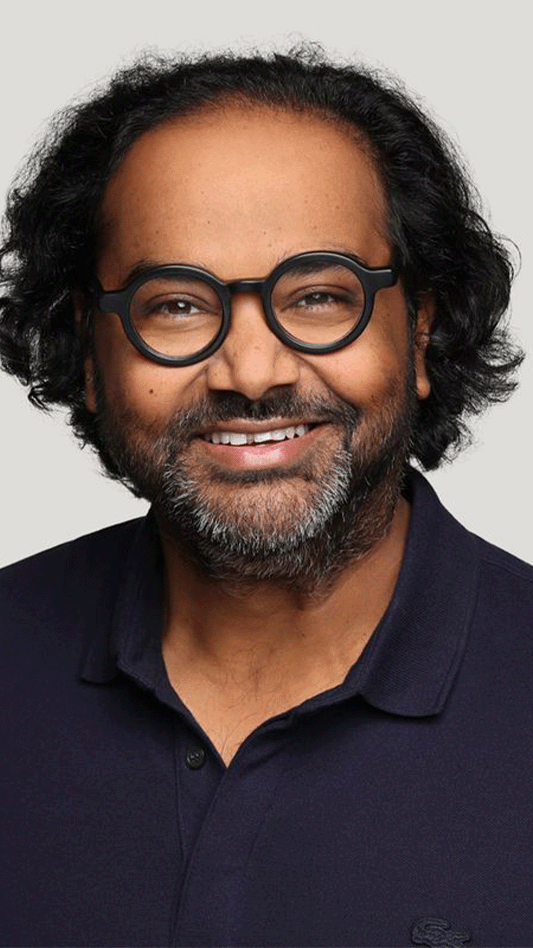
These EPR bills are some of the first pieces of legislation in the U.S. that will require actual counts of materials and weight to measure recyclability. However, manual auditing of these numbers won’t work at scale, and without access to accurate material stream data, material recovery facilities (MRFs) won’t be able to guarantee that all eco-friendly packaging is accurately sorted.
In addition, fast-moving consumer goods producers won’t receive insights into the real-world recyclability of their packaging, and regulators won’t be able to enforce penalties against those who do not meet new guidelines. To address all of these challenges, we must first tackle the lack of visibility into material flows.
The industry has been flying blind
One of the biggest obstacles hindering legacy recycling practices–and the effective rollout of EPR–is the lack of granular material data. Today, only 1 percent of material is monitored in most recycling facilities, using resource-intensive, manual processes.
Where data does exist, it often provides an incomplete picture. Optical sorters gather useful information, but it's often limited to detailed parameters like polymer type and doesn't account for important factors like food-grade status. In addition, most of the data they collect only accounts for the sorting stage of the recovery process, rather than a holistic picture of everything that enters and eventually leaves a MRF.
In the U.S. alone, this lack of full data visibility, digitization and automation has led to only 5 percent of the 40 million tons of plastic scrap being recycled annually, while 86 percent made its way to landfills. Not only is that a major environmental problem, with landfilled and incinerated materials increasing carbon emissions, but facilities are losing out on $80 billion to $120 billion by not recycling this material.
As a result, many may feel disillusioned with recycling and less motivated to participate in recycling programs. That only exacerbates the challenges faced by the waste management industry. Today’s inefficiencies also contribute to the idea recycling is ineffective or even futile. This skepticism stems from various factors, including reports of recyclable materials ending up in landfills or being shipped overseas for processing, and misconceptions about the limitations of recycling technology.
With this in mind, it’s imperative we address the data gaps that impede recycling efficiency, and actively address misconceptions surrounding recycling effectiveness. That way, the industry can move closer to achieving its goals of sustainability and circularity in waste management.
Using AI to harness the power of material data
While data has been lacking, the U.S. has been forced to improve its own recycling efficacy with technological advancements in recent years.
Fully automated, self-adapting facilities have been built to process skyrocketing material flows and deliver higher-quality materials for recycled packaging that can boost profits for recycling plants. WM, for instance, has estimated that automated equipment can process 30-40 percent more tons than non-automated MRFs utilizing a similar footprint. Meanwhile, older legacy plants have become more open to being retrofitted with new technologies to catch up with the power and revenue potential of next-generation MRFs. Now, as we transition into a new era of AI-led innovation, artificial intelligence is presenting the potential to transform recycling efficacy further within both current and future plants.
By applying AI to recognize waste materials from real-time images captured from cameras installed in MRFs, we now can identify nearly every item in the municipal stream that passes through a MRF. Once captured, that data allows us to analyze the material by its mass in the overall stream, distinguish between polyethylenes (PE), track the material’s financial value and even project its potential greenhouse gas (GHG) emissions. As a result, we not only have access to 100 percent of the material data going through MRFs, but we also can receive actionable insights to refine their operations, streamline recycling efforts, and make the overall process more economically and environmentally viable.
This data-driven recycling approach being fostered by AI is carving out one of the few pathways for EPR success. Without comprehensive and accurate recycling data down to a brand or even stock-keeping unit (SKU) level, packaging producers will struggle to assess the true recyclability of their products. That same data will provide an essential feedback loop as producers go on to design–and redesign–more sustainable packaging to maximize recovery rates.
If anything, these EPR bills are about to highlight to packaging producers, MRFs and regulators that for something to be truly recyclable, it has to be collected, sorted, sent somewhere to be processed into another material and then actually used again. Without the data to track this full postconsumer journey, packaging-led recycling will be as ineffective as the consumer-led recycling that came before it.
Skepticism surrounding recycling is often the result of both viewing and tackling the waste crisis problem in isolation. By utilizing material data to align policy, product design and investment, we can ensure that by the time material arrives at a MRF or recycling plant, it’s supposed to be there.
Ambarish Mitra is the co-founder of Greyparrot, a London-based artificial intelligence recycling facility analytics software developer. For more information, visit here.
More from our latest newsletter
- Origin Materials introduces tethered PET beverage cap
- Plastics Industry Association announces Sustainability Innovation Award winners
- Magnomer coatings prequalified by How2Recycle
- Brightmark plans $950M plastics circularity center for Georgia
- Revolution launches trash bags made with PCR
- A plastic film partnership
- WM upgrades its Germantown Recycling Facility in Wisconsin
- CAA moving forward with EPR implementation in Colorado